Decoding Autism: Can MRIs Provide Answers?
April 29, 2024
Unveiling the potential of MRI in autism diagnosis. Can MRIs provide answers about autism? Find out now!
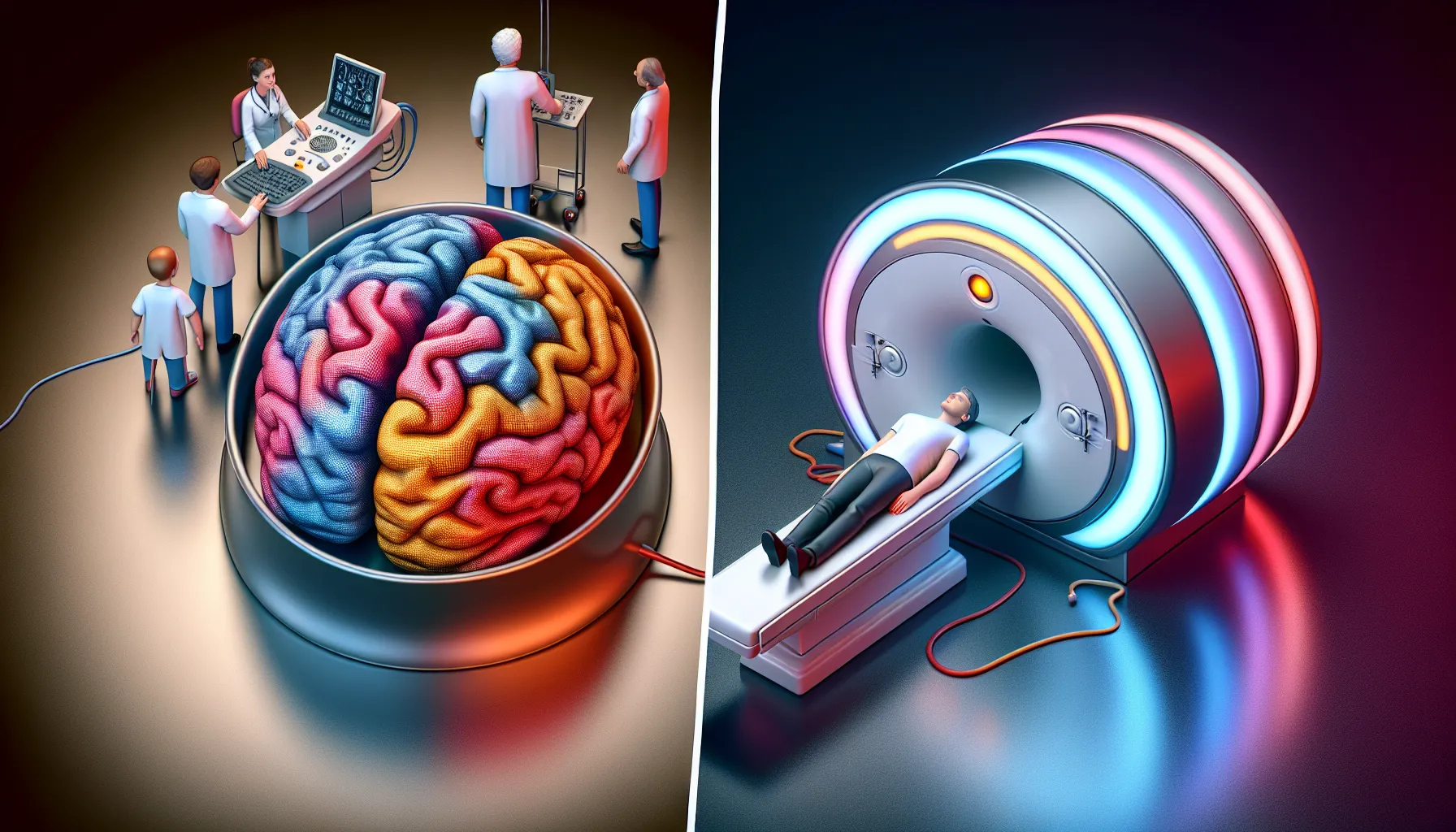
Understanding Autism and MRIs
Autism, a complex neurodevelopmental disorder, can be better understood through the use of Magnetic Resonance Imaging (MRI) scans. MRIs provide valuable insights into the structural and functional abnormalities in the brains of individuals with Autism Spectrum Disorder (ASD). In this section, we will explore the role of functional MRI (fMRI) in autism diagnosis and the abnormalities that can be observed in MRI scans of children with ASD.
Role of FMRI in Autism Diagnosis
Functional MRI (fMRI) plays a significant role in the diagnosis of autism. By analyzing brain activation patterns related to social interactions in individuals with high-functioning autism and neurotypical individuals, fMRI brain scans can diagnose autism with 97% accuracy. This non-invasive imaging technique helps researchers understand how different regions of the brain are activated and connected during various tasks or in resting states. It provides valuable insights into the neural mechanisms underlying social and communication deficits commonly observed in individuals with ASD.
MRI Abnormalities in Children with ASD
Routine magnetic resonance imaging (MRI) scans have been conducted on children with autism spectrum disorder (ASD), revealing certain abnormalities. A study conducted on 117 children with ASD under 5 years old found that 55% of them had abnormal MRI findings. These abnormalities included white matter signal intensity abnormalities, ventricular anomalies, and mega cisterna magna. It is important to note that these abnormalities were more prevalent in the high-functioning subgroup of children with ASD compared to typically developing children.
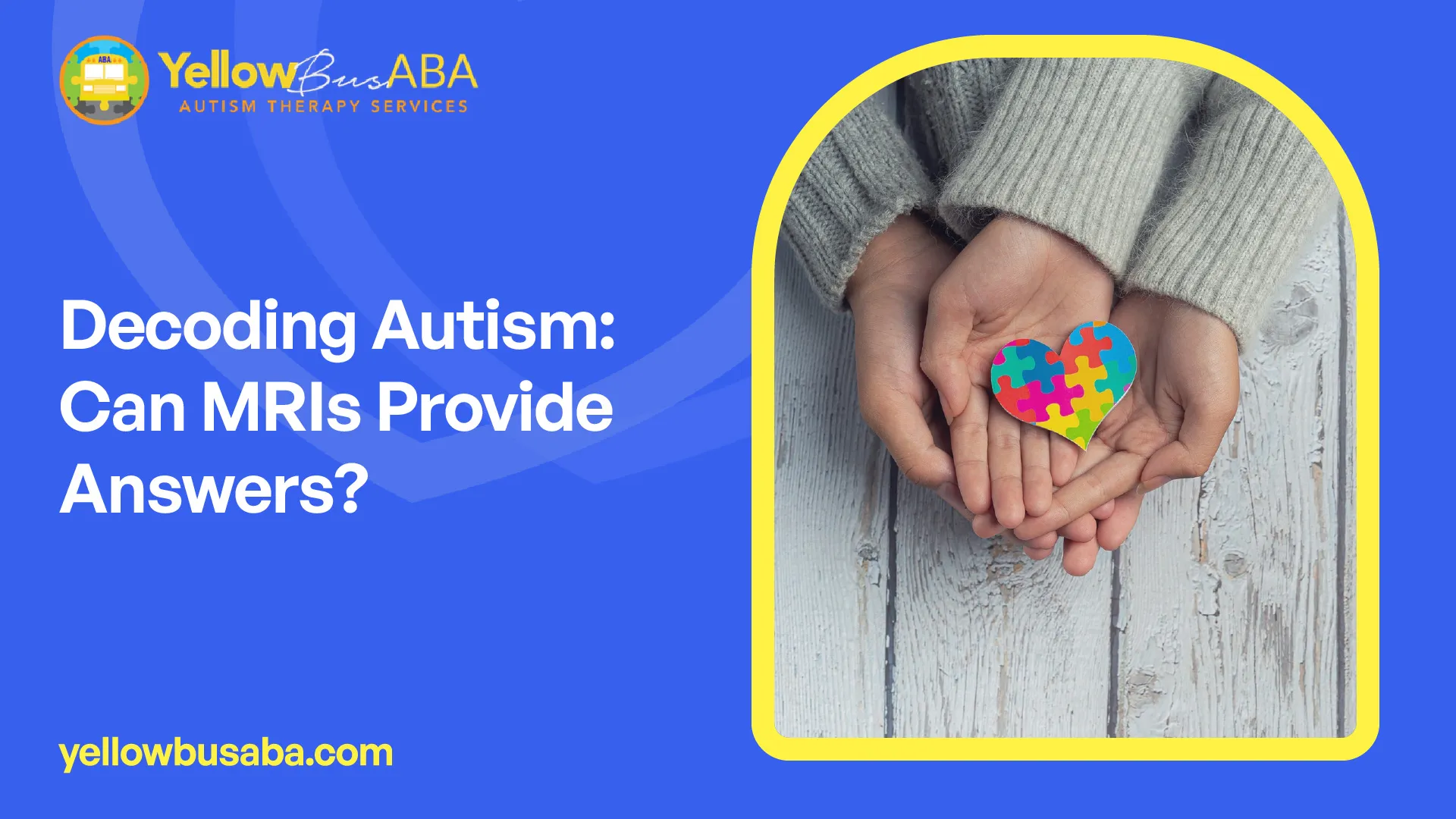
Increased ventricular volume is one of the most replicated findings in individuals with ASD. In fact, it has been reported as a potentially predictive factor for autism diagnosis. Ventricular volume was found to be significantly increased in individuals with ASD compared to typically developing individuals. This finding further supports the role of MRI in providing insights into the structural differences in the brains of individuals with ASD.
Structural MRI examination allows researchers to noninvasively examine brain anatomy in children with ASD. It is widely used to investigate brain morphology due to its high contrast sensitivity and spatial resolution. By analyzing structural abnormalities in the brain, researchers can gain a better understanding of the neural basis of autism.
MRI scans have proven to be valuable tools in understanding the structural and functional abnormalities associated with autism. They provide insights into the neural mechanisms underlying the social and communication deficits observed in individuals with ASD. These findings contribute to early diagnosis and intervention strategies, promoting better outcomes for individuals with autism.
MRI Findings in Autism
Magnetic resonance imaging (MRI) has proven to be a valuable tool in understanding the neurobiological basis of autism spectrum disorder (ASD). By examining the brain's structure and function, MRI studies have provided insights into the underlying mechanisms of ASD. In this section, we will explore some of the key findings from MRI studies in individuals with autism.
Ventricular Volume and ASD
One of the most replicated findings in individuals with ASD is increased ventricular volume. Several studies have reported a higher prevalence of ventricular abnormalities in individuals with ASD compared to typically developing individuals. Increased ventricular volume has been found to be statistically significant and even a potentially predictive factor for the diagnosis of ASD.
Ventricular enlargement in ASD may be indicative of alterations in brain development and connectivity. However, it is important to note that not all individuals with ASD exhibit ventricular abnormalities, and the presence of ventricular enlargement alone is not sufficient for a diagnosis.
Structural MRI in ASD Research
Structural MRI examination allows researchers to noninvasively examine brain anatomy in individuals with ASD. This technique is widely used to investigate brain morphology due to its high contrast sensitivity and spatial resolution. Studies utilizing structural MRI have consistently reported abnormalities in various brain regions in individuals with ASD.
Abnormalities have been observed in the corpus callosum, prefrontal white matter, cingulate gyrus, and internal capsule. These findings suggest disruptions in neural connectivity and communication between different brain regions in individuals with ASD.
Voxel-based Studies in Autism
Voxel-based studies using MRI have further contributed to our understanding of ASD. Voxel-based morphometry (VBM) studies have identified both increased gray matter volume in the frontal and temporal lobes and decreased gray matter density in these regions in individuals with ASD. These findings suggest complex alterations in brain structure associated with ASD.
VBM studies have also revealed differences in other brain regions, such as the amygdala, hippocampus, and cerebellum, which are involved in social cognition, memory, and motor coordination. These findings emphasize the heterogeneity and complexity of the neural underpinnings of ASD.
MRI studies continue to uncover valuable insights into the neurobiology of ASD. However, it is important to note that MRI findings alone cannot be used as a diagnostic tool for ASD. These findings provide valuable information for researchers and clinicians, contributing to a better understanding of the brain differences associated with ASD. Further research is needed to fully comprehend the complex relationship between brain structure and the clinical manifestations of ASD.
Automated ASD Diagnosis
The field of automated autism spectrum disorder (ASD) diagnosis has seen significant advancements with the application of artificial intelligence (AI), machine learning (ML) algorithms, and deep learning (DL) architectures. These technologies have shown promise in assisting specialists with the diagnosis of ASD using magnetic resonance imaging (MRI) modalities.
AI in ASD Diagnosis
Artificial intelligence (AI) has played a crucial role in the automated diagnosis of ASD using MRI data. Computer-aided design systems (CADS) based on AI have been developed to assist specialist physicians in diagnosing ASD. These systems utilize AI algorithms to analyze MRI data, helping to streamline the diagnostic process and provide reliable results.
ML Algorithms for ASD Detection
Machine learning (ML) algorithms have been employed to automate the diagnosis of ASD using MRI modalities. Support vector machines (SVM), random forests, and linear discriminant analysis (LDA) are among the ML algorithms commonly used for this purpose. These algorithms learn from labeled MRI data to identify patterns and classify individuals as either having ASD or not.
DL Architectures for Diagnosis
Deep learning (DL) architectures have also shown promise in the automated diagnosis of ASD using MRI data. Convolutional neural networks (CNNs) and recurrent neural networks (RNNs) are examples of DL architectures that have been applied in this context. DL models learn hierarchies of features from MRI data, enabling accurate classification of individuals with ASD [5].
It is important to note that the development and implementation of automated ASD diagnosis methods face certain challenges. Access to diverse datasets with different types of ASD, as well as suitable hardware resources, are among the challenges encountered in this field. However, the availability of resources such as the ABIDE dataset, which includes fMRI and sMRI data from ASD patients, has contributed to advancements in automated ASD diagnosis.
By harnessing the power of AI, ML algorithms, and DL architectures, researchers and specialists continue to make strides in automating the diagnosis of ASD using MRI modalities. These advancements have the potential to improve the accuracy and efficiency of ASD diagnosis, leading to earlier interventions and improved outcomes for individuals on the autism spectrum.
Advancements in ASD Research
As research into autism spectrum disorder (ASD) progresses, advancements in neuroimaging techniques have provided valuable insights into the structural and functional differences in the brains of individuals with ASD. In this section, we will explore some of the significant advancements in ASD research, including the use of diffusion MRI, conductivity differences in ASD brains, and the role of neuroimaging genetics.
Diffusion MRI in ASD Studies
Diffusion MRI (dMRI) has emerged as a powerful tool in ASD research. This technique measures molecular diffusion in biological tissue, allowing researchers to observe structural differences in the brains of individuals with ASD compared to those without. By examining the microstructural components and their organization, researchers have been able to develop mathematical models that shed light on the etiology of ASD.
One key finding from a study conducted at the University of Virginia is that there is a difference in the diameter of microstructural components in the brains of individuals with autism. These differences can lead to slower conductivity of electrical signals within the brain, affecting its overall function. This groundbreaking research establishes a direct link between the microstructural differences observed in the brains of individuals with ASD and their scores on the Social Communication Questionnaire, a widely used clinical tool for diagnosing ASD.
Conductivity Differences in ASD Brains
Understanding the conductivity of neural axons in the brain is another area of advancement in ASD research. The study conducted by University of Virginia researchers introduces a new metric for understanding ASD by focusing on the physiological differences in brain structures of individuals with ASD as compared to those without. By examining the conductivity of neural axons and their ability to carry information through the brain, researchers have developed a unique approach to calculating neural conductivity.
The study found that individuals with ASD exhibit conductivity differences in their brains compared to typically developing individuals. These differences in neural conductivity provide insights into the origins of ASD and help researchers better understand the neurological underpinnings of the disorder. This novel metric, applied for the first time in a clinical population, holds promise for further advancements in understanding and treating ASD and other neurological conditions.
Neuroimaging Genetics in ASD
Neuroimaging genetics is another significant area of advancement in ASD research. By studying the genetic links to ASD and combining it with neuroimaging data, researchers aim to uncover the complex interplay between genetic factors and brain structure or function. This multidisciplinary approach provides valuable insights into the underlying mechanisms of ASD, potentially leading to improved diagnostic tools and targeted interventions.
By examining the genetic mutations associated with ASD and their impact on brain changes, researchers can identify specific metabolic pathways that may contribute to the development of ASD. This knowledge can help in the development of personalized treatment approaches and interventions tailored to individuals with ASD.
In summary, advancements in ASD research have allowed for a deeper understanding of the condition through techniques such as diffusion MRI, the study of conductivity differences in ASD brains, and the integration of neuroimaging genetics. These advancements bring us closer to unraveling the complex nature of ASD and pave the way for improved diagnostic methods and targeted interventions for individuals on the autism spectrum.
Genetic Links to ASD
The field of autism research has made significant progress in understanding the genetic basis of Autism Spectrum Disorder (ASD). Genetic mutations and alterations have been found to play a crucial role in the development and manifestation of ASD. In this section, we will explore the genetic links to ASD and how they contribute to brain changes in individuals with the disorder.
Genetic Mutations and Brain Changes
Several genes have been associated with structural and functional changes in the brains of individuals with ASD. Mutations in these genes disrupt neuronal development, synaptic functioning, and connectivity in different brain regions. Some of the genes implicated in ASD include Homeobox (HOX) genes, PTEN, SHANK3, CNTNAP2, MET receptor tyrosine kinase, CHD8, and NLGN3.
These genetic mutations can lead to alterations in brain circuitry, affecting the normal development and functioning of neural circuits. For example, mutations in PTEN, SHANK3, and CNTNAP2 have been associated with changes in brain connectivity and synaptic functioning in individuals with ASD. These alterations contribute to the core symptoms of ASD.
Metabolic Pathways in ASD
In addition to genetic mutations, researchers have also explored the role of metabolic pathways in ASD. Metabolic dysregulation has been observed in individuals with ASD, suggesting a potential link between metabolism and the disorder. However, the exact mechanisms underlying this relationship are still being investigated.
Understanding the metabolic pathways involved in ASD can provide insights into the biological processes that contribute to the disorder. Further research is needed to uncover the specific metabolic alterations and their impact on brain function in individuals with ASD.
Neuroimaging Genetics Studies
Neuroimaging genetics studies have emerged as a valuable approach in ASD research. These studies integrate neuroimaging techniques with genomic approaches to identify associations between genetic variants and brain morphology and function in individuals with ASD.
By combining neuroimaging data with genetic information, researchers can refine the understanding of how specific genetic variants influence brain structure and function in ASD. This approach allows for the localization of genetic effects to specific tissue layers and provides insights into the complex interactions between genes, brain structure, and function.
Large sample sizes and advanced methods are crucial for examining gene-gene and gene-environment interactions in ASD. The integration of neuroimaging and genetics holds promise for identifying diagnostic biomarkers and understanding the underlying biological mechanisms of ASD.
The genetic links to ASD provide valuable insights into the biological basis of the disorder. Genetic mutations and alterations contribute to brain changes and disruptions in neuronal development and connectivity. Further research in neuroimaging genetics can enhance our understanding of ASD and potentially lead to the development of novel diagnostic biomarkers and targeted interventions.
ML and DL Application
In the field of autism research, machine learning (ML) and deep learning (DL) techniques have shown promise in their application to neuroimaging data for the diagnosis of Autism Spectrum Disorder (ASD). By leveraging advanced algorithms, these methods can extract features from structural and functional brain data, aiding in the classification of ASD and the identification of objective biomarkers.
Predictive Models for ASD Diagnosis
ML algorithms, such as support vector machines (SVM), random forests, and linear discriminant analysis (LDA), have been utilized for the development of predictive models in the diagnosis of ASD using MRI modalities. These models are trained on a large dataset of neuroimaging data, allowing them to learn patterns and make predictions based on new input.
Similarly, DL architectures, including convolutional neural networks (CNNs) and recurrent neural networks (RNNs), have been employed for automated diagnosis of ASD using MRI modalities. These architectures are capable of learning complex representations of neuroimaging data, enabling them to make accurate predictions about the presence of ASD.
Unsupervised ML in ASD Research
Unsupervised ML methods, such as hierarchical clustering and k-means clustering, have demonstrated utility in predicting short-term outcomes and exploring cluster-based treatment efficacy in ASD. These methods allow researchers to identify subgroups within the ASD population, leading to a better understanding of the heterogeneity of the disorder and potentially guiding personalized treatment approaches.
Feature Extraction in Neuroimaging
ML and DL techniques excel at extracting meaningful features from neuroimaging data. By analyzing structural and functional brain images, these methods can identify patterns and relationships that may not be readily apparent to human observers. This feature extraction process plays a crucial role in the development of accurate predictive models for ASD diagnosis.
Through the application of ML and DL techniques to neuroimaging data, researchers aim to improve the accuracy and efficiency of ASD diagnosis. These advanced methods have the potential to identify objective biomarkers and provide valuable insights into the underlying neurobiological mechanisms of ASD. As research in this field continues to advance, ML and DL hold promise for enhancing our understanding of ASD and improving diagnostic practices.
References
- https://time.com/3614487/fmri-autism-diagnosis/
- https://www.ncbi.nlm.nih.gov/pmc/articles/PMC7602717/
- https://www.ncbi.nlm.nih.gov/pmc/articles/PMC10210579/
- https://www.ncbi.nlm.nih.gov/pmc/articles/PMC3081653/
- https://www.ncbi.nlm.nih.gov/pmc/articles/PMC9577321/
- https://www.sciencedaily.com/releases/2024/04/240417182844.htm
- https://www.nature.com/articles/s41380-023-02060-9